Analytics
Demystifying Artificial Intelligence and Machine Learning
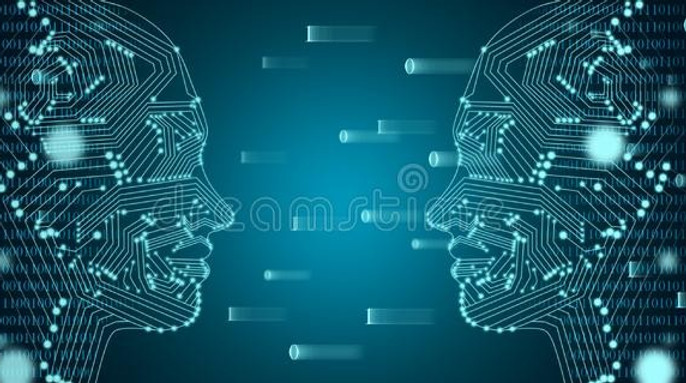
There have always been tech buzz words that people feel the need to throw into casual conversation in order to feel smart, current and relevant. A decade ago it was Big Data, more recently it was the Cloud, and today it is Artificial Intelligence (aka AI) and Machine Learning (aka ML) both used interchangeably and often incorrectly. This is a short article to help those who would like to have a clearer understanding of what AI and ML are, how and where they are used, and where it doesn’t make sense to use them.
What is Artificial Intelligence?
The dictionary defines AI as the simulation of human intelligence in machines that are programmed to think like humans and mimic their actions. In business today, AI is an embedded function within software and applications used to automate repetitive tasks. When you understand that it is really a way to automate repetitive tasks, it doesn’t seem all that intelligent. Examples of common applications of AI include:
-
Recommendation engines
-
Chatbots
-
Personalization
-
HR recruiting
In short, if the data exists, the task is repeatable and there are means for leveraging the outcome, AI will improve productivity.
What is Machine Learning?
ML is a subset of AI where algorithms improve the predictive outcomes as more data becomes available. Models are developed on an initial data set and then over time are optimized as more data is input. The examples of AI above are also examples of ML, but whereas AI streamlines productivity, ML improves decision making over time. Specific examples of ML are:
-
Pandora’s recommendation engine which improves the suggested music as the user provides feedback on the songs that are playing within the selected genre.
-
Netflix’s recommendation engine is similar to Pandora’s, as it recommends new shows and movies based on what was recently watched. They go a step further and present the percent match of any show that is clicked on to review.
-
Instagram’s advertising model is based not only upon the user’s activity on Instagram itself, but on any other third-party site and app. As a result, the ads you see as you scroll through your Instagram feed are very often correlated to the things you’ve most recently Googled, websites you’ve recently visited, even the things you say out loud in conversation. As Vice describes, the apps we use daily “are designed with audible ‘triggers’ that tell the app to record and store data,” leading to the freaky experience of seeing an advertisement for the exact product you just casually mentioned in conversation with your friend.
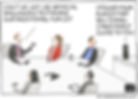
What AI/ML are not...
As the title of this piece alludes to, there is a level of mystery around artificial intelligence and machine learning. Beyond that is also an element of fear, concern, or even danger; perhaps we can thank the Sci-Fi genre for hypothetical scenarios where robots gain ultimate domination. Nevertheless, AI and ML are not poised to take over our world or our computers. The crux behind most of the misunderstandings of AI are that it operates autonomously, when in reality it is completely dependent on the data input by humans. In fact, the more data humans can provide, the more effective and ‘intelligent’ the technology will be.
Also, AI and ML do not replace traditional analytics where an analysts and data scientists look at the meaning of the information. Data interpretation and story telling are important analytic steps which AI and ML do not do. The example in Amy’s “Flying Blind in a Time of Crisis: How Analytics Can Help us Thrive” demonstrates the importance of these steps. Another way to look at it is that AI and ML actually support analysts by eliminating some of behind the scenes grunt work so that they can focus on the interpretation, education and applications which are much more rewarding.
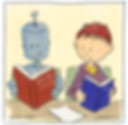
When they do not work...
AI and ML work as long as the organization has the data to input and the ability to leverage the output. ML needs a lot of data to be successful. Another application of ML is Spotify, where new songs and artists are recommended based on your playlists. But what happens when the data available isn’t consistent? Amy’s son, his girlfriend and she share a Spotify account. They are into rap and Amy prefers classic and alternative rock. Spotify typically recommends funk and punk rock based on their combined preferences as opposed to the respective rap and classic rock recommendations the individuals would prefer to see.
Returning to the advertising phenomenon of devices picking up on “triggers” and populating ads accordingly, things can get a little confused when there is more nuance in conversation. For example, Verity spent time looking at swimsuits online in the beginning of the summer and immediately began seeing ads for youthful, summertime apparel. Simultaneously, she had conversations with her mother, an Occupational Therapist, around various braces and therapeutic solutions for stroke patients and people with arthritis. Verity began seeing ads for these types of products and has received further advertising targeted towards the senior population, leaving her apps a bit jumbled up as to what demographic she actually fits into.
More Advanced Applications of AI: Looking Forward
At this point we’ve hopefully clarified some of the basics around AI and ML, and discussed largely their limitations. This should serve as assurance that people won’t be losing their jobs to be replaced by machines. However, history has proven that technology continues to advance; the applications of artificial intelligence and machine learning are certainly expanding in ways that will augment human teams and create time for more mission critical work.
Example: A certain cybersecurity firm has applied unsupervised machine learning to develop technology that can imitate the work of the firm’s own human analyst team in serving network analyses to their IT clients. The analyses are built upon the findings produced by the firm’s flagship product, which is also AI-driven. Through constantly ingesting network traffic, the flagship technology has the amount of data required to produce meaningful insights and become nuanced through machine learning. The same unsupervised machine learning was used to study the behavior of human analyst activity in troubleshooting cyber events, so that the technology is now competent enough to analyze without human input.
The takeaway is not that humans are now extraneous; it is that their capabilities can be supplemented by technology to open doors for more creative and original work. The future is bright!
In Summary
AI and ML are valuable technologies that streamline and enhance the work that analysts and data scientists do. On the flip side, you can see how AI and ML can easily lead to misinformation given data input or if the output is taken out of context. Like all high-performance machines, they need to be driven by people who understand their strengths and limitations, and can provide the overlay and context that, thankfully for the human race, only humans can provide. If you are interested in leveraging AI or ML gainfully but are not sure if you and your company are ready, Zapfel Group can help.